From Logs to Salience: Real-Time Ingestion with Attixa
In today's fast-paced digital environment, the ability to process and make sense of live data streams is crucial. Traditional batch processing approaches often fail to capture the dynamic nature of real-time information. This article explores how Attixa's real-time ingestion system transforms streaming data into meaningful, contextually relevant memories.
The Challenge of Real-Time Data
Processing live data streams presents several unique challenges:
- Volume: Handling high-throughput data streams
- Velocity: Processing information as it arrives
- Variety: Managing different data formats and structures
- Veracity: Ensuring data quality and relevance
- Value: Extracting meaningful insights in real-time
Attixa's Real-Time Processing Pipeline
Here's how Attixa handles real-time data ingestion:
from attixa import StreamProcessor
# Initialize the stream processor
processor = StreamProcessor(
config={
"window_size": "5m",
"salience_threshold": 0.7,
"context_preservation": True
}
)
# Define processing pipeline
pipeline = processor.create_pipeline(
steps=[
"normalize",
"enrich",
"analyze",
"store"
]
)
# Process streaming data
async def process_stream(stream):
async for event in stream:
# Normalize and enrich the data
processed = await pipeline.process(event)
# Store with contextual metadata
await processor.store(
content=processed["content"],
context={
"source": processed["source"],
"timestamp": processed["timestamp"],
"importance": processed["salience_score"]
}
)
Key Components of Real-Time Processing
- Stream Normalization
# Normalize incoming data
normalized = await processor.normalize(
data=raw_event,
schema={
"required_fields": ["timestamp", "content", "source"],
"optional_fields": ["metadata", "tags"]
}
)
- Context Enrichment
# Enrich with additional context
enriched = await processor.enrich(
data=normalized,
context_sources=[
"user_profile",
"system_state",
"temporal_context"
]
)
- Salience Analysis
# Analyze importance and relevance
analysis = await processor.analyze(
data=enriched,
metrics=[
"temporal_relevance",
"contextual_importance",
"relationship_strength"
]
)
Implementation Patterns
Here are common patterns for implementing real-time ingestion:
- Event Processing
# Process individual events
async def process_event(event):
# Extract key information
content = event["content"]
context = event["context"]
# Calculate salience
salience = await processor.calculate_salience(
content=content,
context=context
)
# Store if sufficiently important
if salience > processor.config["salience_threshold"]:
await processor.store(content, context)
- Batch Processing
# Process events in batches
async def process_batch(events):
# Group by time window
windowed = processor.window(events, size="5m")
# Process each window
for window in windowed:
# Calculate aggregate salience
aggregate = await processor.aggregate_salience(window)
# Store important patterns
if aggregate["importance"] > threshold:
await processor.store_pattern(window)
- State Management
# Maintain processing state
class ProcessingState:
def __init__(self):
self.current_window = []
self.aggregate_context = {}
async def update(self, event):
# Update window
self.current_window.append(event)
# Update context
self.aggregate_context.update(event["context"])
# Process if window full
if len(self.current_window) >= window_size:
await self.process_window()
Best Practices for Real-Time Processing
-
Configuration
- Set appropriate window sizes
- Define salience thresholds
- Configure context preservation
-
Monitoring
- Track processing latency
- Monitor memory usage
- Alert on anomalies
-
Optimization
- Balance batch size and latency
- Optimize storage patterns
- Manage resource usage
Real-World Applications
Attixa's real-time ingestion system is being used in various scenarios:
- Log Analysis: Processing and analyzing system logs in real-time
- User Behavior: Tracking and understanding user interactions
- Market Data: Processing and analyzing financial market data
- IoT Streams: Handling sensor data from IoT devices
The Future of Real-Time Processing
As data streams continue to grow in volume and complexity, the need for sophisticated real-time processing will only increase. Attixa's approach to real-time ingestion provides a foundation for building more responsive and intelligent systems.
Ready to process your data streams in real-time? Check out our documentation or try our stream processing quickstart.
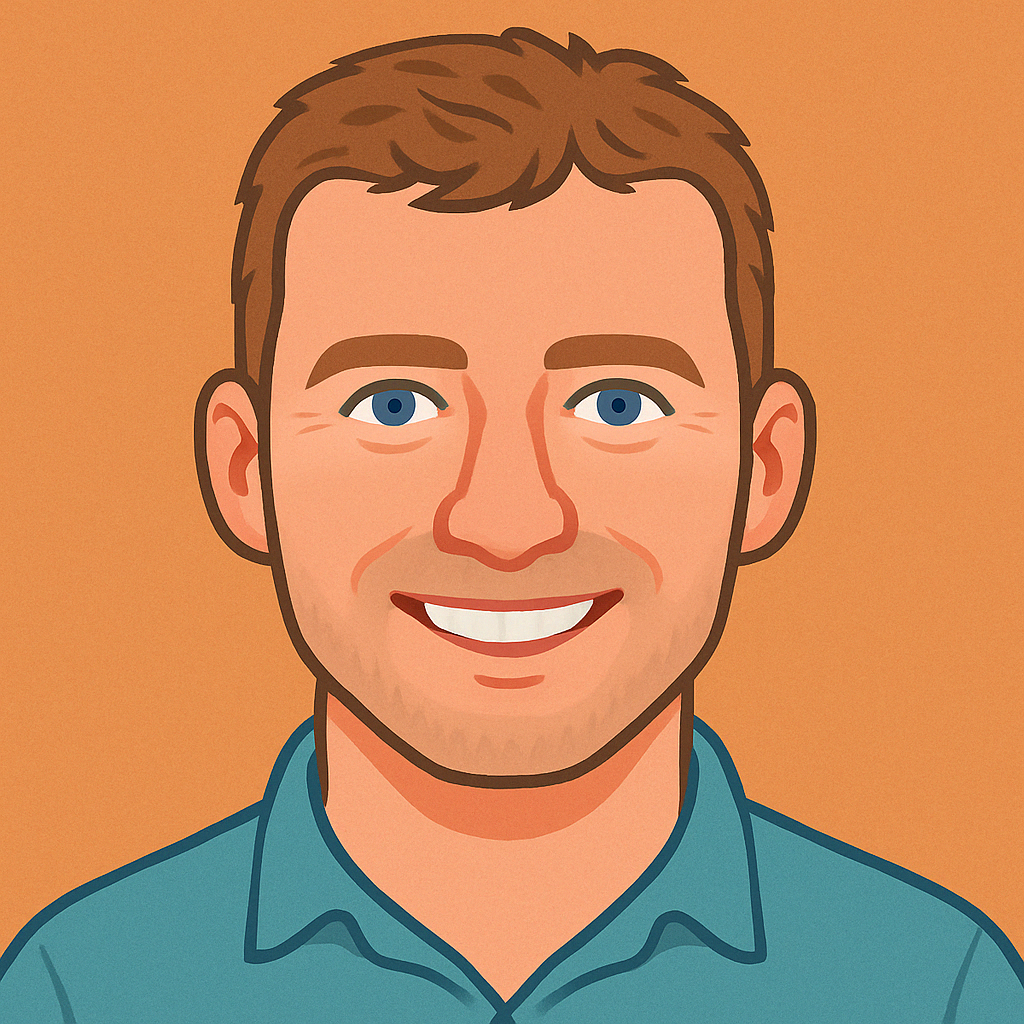
Allan Livingston
Founder of Attixa
Allan is the founder of Attixa and a longtime builder of AI infrastructure and dev tools. He's always dreamed of a better database ever since an intern borrowed his favorite DB systems textbook, read it in the bathroom, and left it on the floor. His obsession with merging database paradigms goes way back to an ill-advised project to unify ODBC and hierarchical text retrieval. That one ended in stack traces and heartbreak. These scars now fuel his mission to build blazing-fast, salience-aware memory for agents.