Building a Chatbot That Actually Remembers Stuff with Attixa
Most chatbots today suffer from amnesia - they forget conversations as soon as they end, leading to frustrating user experiences. This guide shows how to build a chatbot with real memory using Attixa, creating more natural and helpful interactions.
The Memory Problem in Chatbots
Traditional chatbots face several memory-related challenges:
- Context Loss: Conversations reset after each message
- Personalization Gaps: No memory of user preferences
- Repetition: Same questions asked repeatedly
- Inconsistency: Responses vary without context
How Attixa Solves These Problems
Attixa's memory system provides:
- Persistent Memory: Store and retrieve conversation history
- Contextual Understanding: Maintain conversation context
- Personalization: Remember user preferences and patterns
- Consistency: Ensure coherent responses over time
Building the Chatbot
Here's how to create a memory-enabled chatbot:
from attixa import MemorySystem
from attixa.chat import Chatbot
# Initialize Attixa and chatbot
memory = MemorySystem()
chatbot = Chatbot(
memory_system=memory,
personality="helpful assistant"
)
# Basic conversation
async def chat(message, user_id):
# Get conversation history
history = await chatbot.get_history(user_id)
# Get relevant context
context = await chatbot.get_context(
message=message,
history=history
)
# Generate response
response = await chatbot.generate_response(
message=message,
context=context
)
# Store interaction
await chatbot.store_interaction(
user_id=user_id,
message=message,
response=response,
context=context
)
return response
Advanced Memory Features
- Conversation Memory
async def handle_conversation(user_id, message):
# Get recent conversations
recent = await chatbot.get_recent_conversations(
user_id=user_id,
limit=5
)
# Get relevant memories
memories = await chatbot.get_relevant_memories(
message=message,
user_id=user_id
)
# Combine context
context = await chatbot.combine_contexts(
recent=recent,
memories=memories
)
return context
- Personalization
async def personalize_response(user_id, response):
# Get user preferences
preferences = await chatbot.get_user_preferences(user_id)
# Get interaction patterns
patterns = await chatbot.get_user_patterns(user_id)
# Personalize response
personalized = await chatbot.personalize(
response=response,
preferences=preferences,
patterns=patterns
)
return personalized
- Memory Management
async def manage_memory(user_id):
# Clean up old conversations
await chatbot.cleanup_old_conversations(
user_id=user_id,
max_age=timedelta(days=30)
)
# Update salience scores
await chatbot.update_salience(
user_id=user_id,
interaction_type="conversation"
)
# Compress frequent patterns
await chatbot.compress_patterns(user_id)
Best Practices
-
Memory Management
- Set appropriate retention periods
- Implement cleanup routines
- Monitor memory usage
- Optimize storage
-
Context Handling
- Maintain conversation boundaries
- Preserve important context
- Clean up irrelevant information
- Balance context length
-
Personalization
- Respect user privacy
- Allow preference updates
- Handle edge cases
- Monitor effectiveness
Real-World Examples
Here are some practical applications:
- Customer Support: Maintain context across tickets
- Personal Assistants: Remember user preferences
- Educational Bots: Track learning progress
- Therapeutic Chatbots: Maintain session context
Next Steps
Ready to build your memory-enabled chatbot? Check out our documentation or try our chatbot guide.
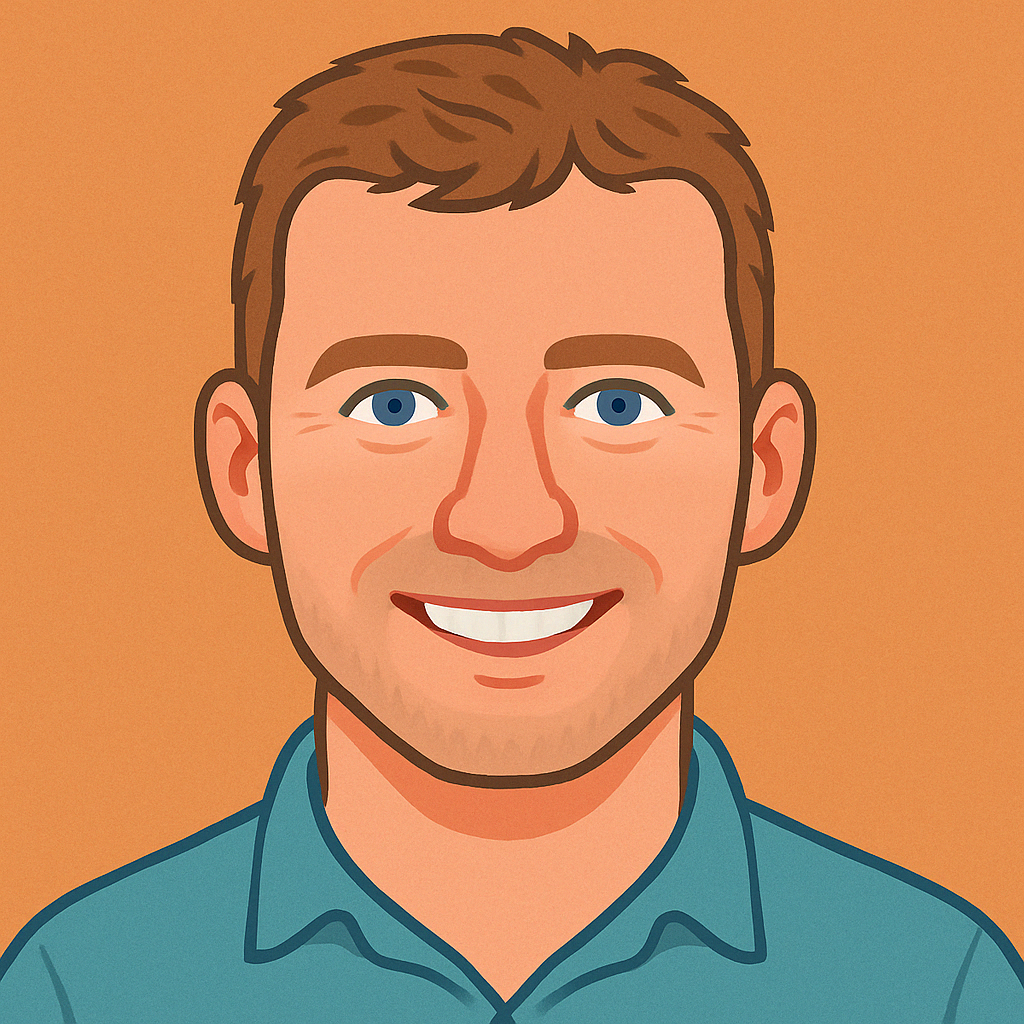
Allan Livingston
Founder of Attixa
Allan is the founder of Attixa and a longtime builder of AI infrastructure and dev tools. He's always dreamed of a better database ever since an intern borrowed his favorite DB systems textbook, read it in the bathroom, and left it on the floor. His obsession with merging database paradigms goes way back to an ill-advised project to unify ODBC and hierarchical text retrieval. That one ended in stack traces and heartbreak. These scars now fuel his mission to build blazing-fast, salience-aware memory for agents.