Attixa + Qdrant + OpenAI = 🔥: A Full RAG Stack in 20 Minutes
Building a robust RAG system requires careful integration of multiple components. This guide shows how to combine Attixa's memory capabilities with Qdrant's efficient vector search and OpenAI's powerful generation to create a production-ready RAG stack in minutes.
Why This Stack?
Each component brings unique strengths:
- Attixa: Sophisticated memory and context management
- Qdrant: Fast and scalable vector similarity search
- OpenAI: State-of-the-art text generation
- Together: A complete, production-ready RAG solution
Quick Start Implementation
Here's how to set up the full stack:
from attixa import MemorySystem
from qdrant_client import QdrantClient
from openai import OpenAI
from attixa.rag import RAGSystem
# Initialize components
memory = MemorySystem()
qdrant = QdrantClient("localhost", port=6333)
openai_client = OpenAI(api_key="your-api-key")
# Create RAG system
rag = RAGSystem(
memory_system=memory,
vector_store=qdrant,
llm=openai_client
)
# Use the system
response = await rag.query(
"What are the best practices for RAG systems?",
context={
"domain": "AI",
"recency": "last 6 months"
}
)
Core Components Integration
- Document Processing Pipeline
async def process_documents(documents):
# Split documents
chunks = await rag.split_documents(documents)
# Generate embeddings
embeddings = await rag.generate_embeddings(chunks)
# Store in Qdrant
await rag.vector_store.upsert(
points=[
{
"id": chunk.id,
"vector": embedding,
"payload": {
"text": chunk.text,
"metadata": chunk.metadata
}
}
for chunk, embedding in zip(chunks, embeddings)
]
)
# Store in Attixa
await rag.memory_system.store(
content=chunks,
context={
"source": "document_processing",
"timestamp": datetime.now()
}
)
- Query Processing
async def process_query(query, context=None):
# Generate query embedding
query_embedding = await rag.generate_embeddings([query])[0]
# Search in Qdrant
vector_results = await rag.vector_store.search(
query_vector=query_embedding,
limit=5
)
# Get context from Attixa
memory_context = await rag.memory_system.retrieve(
query=query,
filters=context
)
# Combine results
combined_context = await rag.combine_contexts(
vector_results=vector_results,
memory_context=memory_context
)
# Generate response
response = await rag.generate_response(
query=query,
context=combined_context
)
return response
- Memory Integration
async def update_memory(query, response, context):
# Store interaction
await rag.memory_system.store(
content={
"query": query,
"response": response,
"context": context
},
metadata={
"type": "interaction",
"timestamp": datetime.now()
}
)
# Update salience scores
await rag.memory_system.update_salience(
content_ids=[result.id for result in context["vector_results"]],
interaction_type="query_response"
)
Advanced Features
- Hybrid Search
async def hybrid_search(query):
# Vector search
vector_results = await rag.vector_store.search(
query_vector=await rag.generate_embeddings([query])[0],
limit=3
)
# Keyword search
keyword_results = await rag.memory_system.keyword_search(
query=query,
limit=3
)
# Combine results
return await rag.rank_results(
vector_results=vector_results,
keyword_results=keyword_results,
weights=[0.6, 0.4]
)
- Contextual Reranking
async def rerank_results(results, query, context):
# Get memory context
memory_context = await rag.memory_system.retrieve(
query=query,
filters=context
)
# Rerank based on memory
return await rag.rerank(
results=results,
context=memory_context,
scoring_function="relevance_with_memory"
)
- Feedback Loop
async def process_feedback(query, response, feedback):
# Update memory based on feedback
await rag.memory_system.store(
content={
"query": query,
"response": response,
"feedback": feedback
},
context={
"type": "feedback",
"timestamp": datetime.now()
}
)
# Adjust salience scores
await rag.memory_system.adjust_salience(
content_ids=[result.id for result in response["context"]],
feedback_score=feedback["score"]
)
Best Practices
-
System Configuration
- Optimize chunk sizes
- Set appropriate limits
- Configure caching
- Monitor performance
-
Memory Management
- Implement cleanup routines
- Set retention policies
- Monitor memory usage
- Optimize storage
-
Performance Optimization
- Use batch processing
- Implement caching
- Optimize queries
- Monitor latency
Real-World Examples
Here are some production use cases:
- Knowledge Bases: Enterprise documentation
- Customer Support: Automated assistance
- Research Tools: Academic literature
- Content Creation: Writing assistance
Next Steps
Ready to build your RAG stack? Check out our documentation or try our quickstart guide.
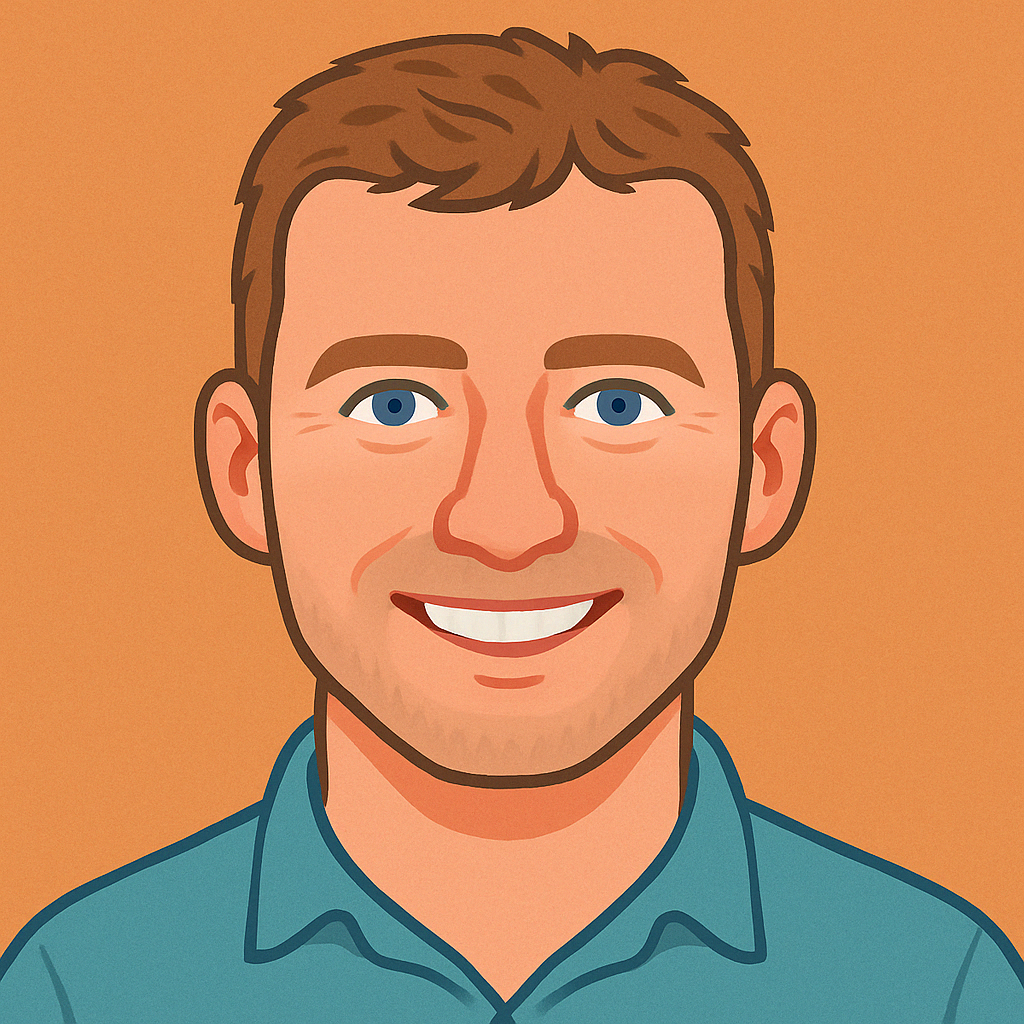
Allan Livingston
Founder of Attixa
Allan is the founder of Attixa and a longtime builder of AI infrastructure and dev tools. He's always dreamed of a better database ever since an intern borrowed his favorite DB systems textbook, read it in the bathroom, and left it on the floor. His obsession with merging database paradigms goes way back to an ill-advised project to unify ODBC and hierarchical text retrieval. That one ended in stack traces and heartbreak. These scars now fuel his mission to build blazing-fast, salience-aware memory for agents.