How AI Researchers Can Use Attixa to Prototype New Memory Models
Attixa provides a powerful platform for AI researchers to prototype and test new memory models. This guide shows how to leverage Attixa's flexible architecture to experiment with novel memory approaches and validate research hypotheses.
Research Capabilities
1. Custom Memory Models
# Custom memory model example
class CustomMemoryModel:
def __init__(self, config):
self.config = config
self.base_memory = MemorySystem()
async def store(self, content, context):
# Custom storage logic
processed = await self.preprocess(content)
structured = await self.structure(processed)
await self.base_memory.store(structured, context)
async def retrieve(self, query, context):
# Custom retrieval logic
results = await self.base_memory.search(query)
filtered = await self.filter_results(results, context)
return await self.rank_results(filtered)
2. Experimental Features
# Experimental feature example
class ExperimentalMemory:
def __init__(self):
self.memory = MemorySystem()
self.experimental_features = {
"novel_retrieval": True,
"custom_scoring": True,
"dynamic_adaptation": True
}
async def test_feature(self, feature, data):
# Enable experimental feature
self.experimental_features[feature] = True
# Run experiment
results = await self.run_experiment(data)
# Analyze results
analysis = await self.analyze_results(results)
return analysis
3. Research Tools
# Research tools example
class ResearchTools:
async def analyze_memory(self, content):
# Analyze memory structure
structure = await self.analyze_structure(content)
# Analyze retrieval patterns
patterns = await self.analyze_patterns(content)
# Analyze performance
performance = await self.analyze_performance(content)
return {
"structure": structure,
"patterns": patterns,
"performance": performance
}
Implementation Examples
1. Novel Retrieval Models
# Novel retrieval model example
class NovelRetrieval:
async def retrieve(self, query, context):
# Implement novel retrieval algorithm
candidates = await self.find_candidates(query)
# Apply custom scoring
scored = await self.score_candidates(candidates, context)
# Use novel ranking
ranked = await self.rank_novel(scored)
return ranked
2. Memory Architecture Experiments
# Memory architecture experiment
class ArchitectureExperiment:
async def test_architecture(self, config):
# Initialize test architecture
architecture = await self.initialize_architecture(config)
# Run test scenarios
results = await self.run_scenarios(architecture)
# Analyze performance
analysis = await self.analyze_performance(results)
# Compare with baseline
comparison = await self.compare_with_baseline(analysis)
return comparison
3. Learning Mechanism Research
# Learning mechanism research
class LearningResearch:
async def study_learning(self, data):
# Implement learning mechanism
learning = await self.implement_learning(data)
# Track learning progress
progress = await self.track_progress(learning)
# Analyze learning patterns
patterns = await self.analyze_patterns(progress)
# Evaluate effectiveness
evaluation = await self.evaluate_effectiveness(patterns)
return evaluation
Research Workflow
1. Experiment Setup
# Experiment setup
class ExperimentSetup:
async def setup_experiment(self, config):
# Configure environment
environment = await self.configure_environment(config)
# Prepare data
data = await self.prepare_data(config)
# Initialize metrics
metrics = await self.initialize_metrics()
# Set up logging
logging = await self.setup_logging()
return {
"environment": environment,
"data": data,
"metrics": metrics,
"logging": logging
}
2. Data Collection
# Data collection
class DataCollection:
async def collect_data(self, experiment):
# Collect baseline data
baseline = await self.collect_baseline(experiment)
# Collect experimental data
experimental = await self.collect_experimental(experiment)
# Process data
processed = await self.process_data(baseline, experimental)
return processed
3. Analysis Tools
# Analysis tools
class AnalysisTools:
async def analyze_results(self, data):
# Statistical analysis
stats = await self.statistical_analysis(data)
# Pattern analysis
patterns = await self.pattern_analysis(data)
# Performance analysis
performance = await self.performance_analysis(data)
return {
"statistics": stats,
"patterns": patterns,
"performance": performance
}
Best Practices
1. Experimental Design
- Clear hypotheses
- Controlled variables
- Reproducible setup
- Thorough documentation
2. Data Management
- Organized datasets
- Version control
- Metadata tracking
- Backup systems
3. Analysis
- Statistical rigor
- Pattern recognition
- Performance metrics
- Comparative studies
Real-World Examples
1. Memory Architecture Research
# Memory architecture research
class ArchitectureResearch:
async def study_architecture(self, config):
# Test different architectures
architectures = await self.test_architectures(config)
# Compare performance
comparison = await self.compare_performance(architectures)
# Analyze tradeoffs
tradeoffs = await self.analyze_tradeoffs(comparison)
return tradeoffs
2. Learning Mechanism Studies
# Learning mechanism studies
class LearningStudies:
async def study_learning(self, data):
# Implement learning mechanisms
mechanisms = await self.implement_mechanisms(data)
# Track learning progress
progress = await self.track_progress(mechanisms)
# Analyze effectiveness
effectiveness = await self.analyze_effectiveness(progress)
return effectiveness
3. Retrieval Algorithm Research
# Retrieval algorithm research
class RetrievalResearch:
async def study_retrieval(self, algorithms):
# Test retrieval algorithms
results = await self.test_algorithms(algorithms)
# Compare performance
comparison = await self.compare_algorithms(results)
# Analyze tradeoffs
tradeoffs = await self.analyze_tradeoffs(comparison)
return tradeoffs
Next Steps
Ready to start your research? Check out our research guide or join our research community.
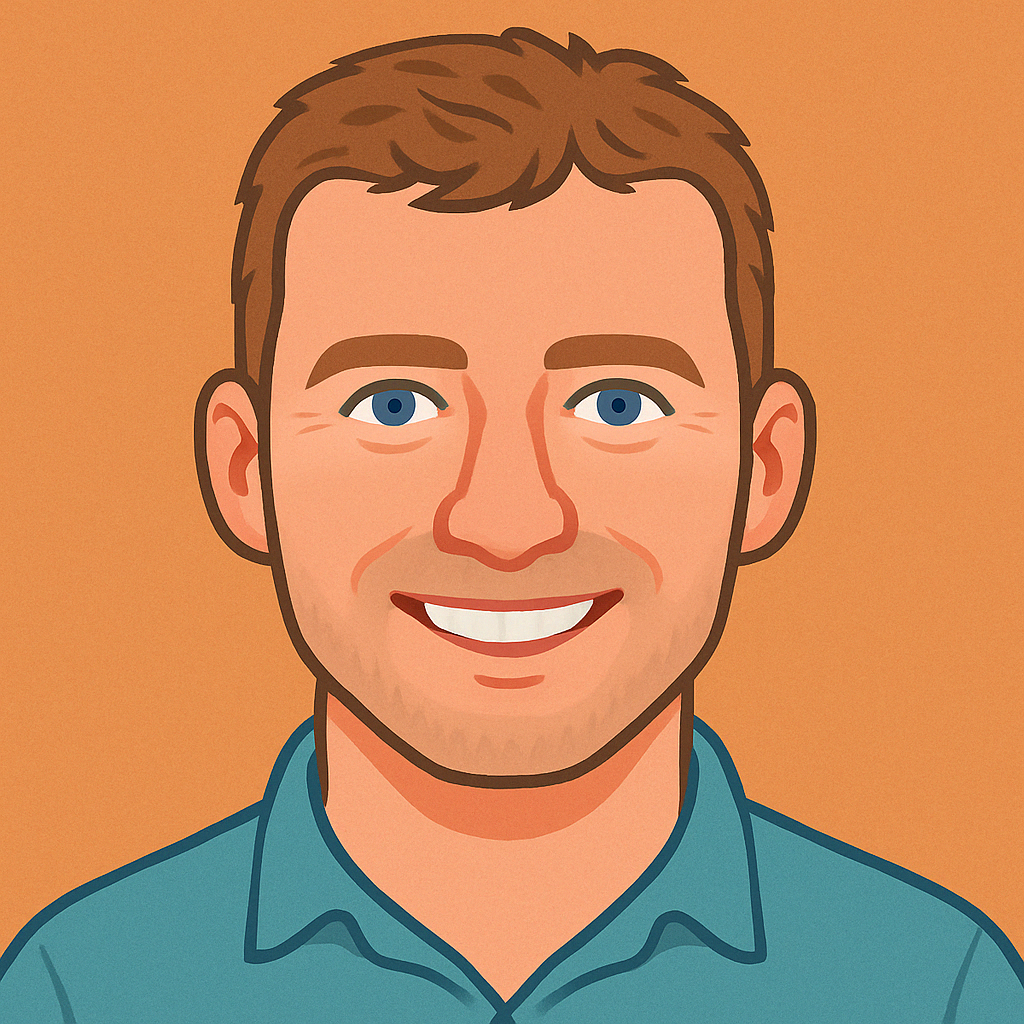
Allan Livingston
Founder of Attixa
Allan is the founder of Attixa and a longtime builder of AI infrastructure and dev tools. He's always dreamed of a better database ever since an intern borrowed his favorite DB systems textbook, read it in the bathroom, and left it on the floor. His obsession with merging database paradigms goes way back to an ill-advised project to unify ODBC and hierarchical text retrieval. That one ended in stack traces and heartbreak. These scars now fuel his mission to build blazing-fast, salience-aware memory for agents.